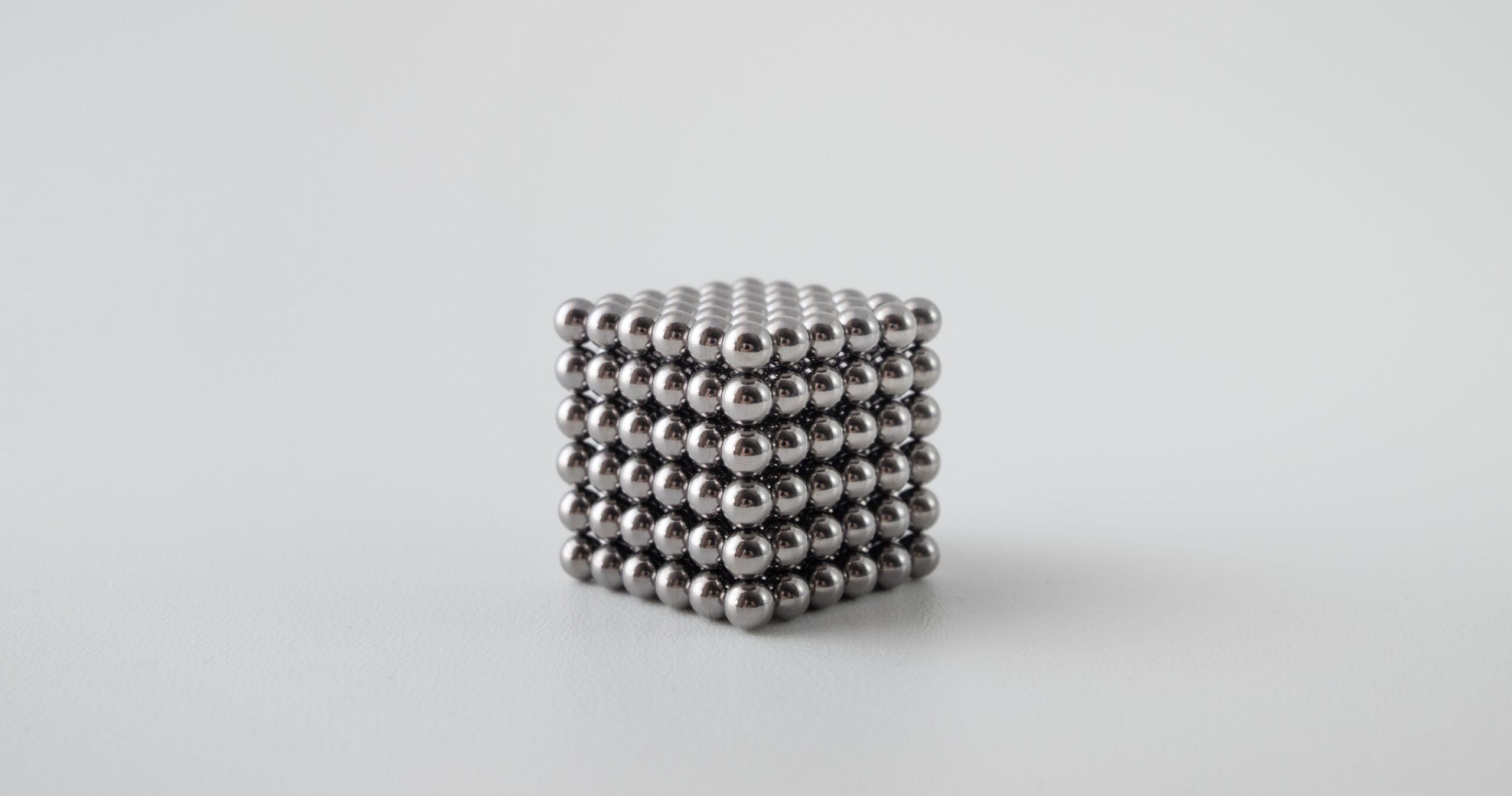
Certain numbers of metal atoms can assemble into stable nanoparticles with symmetrical, crystalline shapes. Even more interesting are the irregular or unstable shapes that arise with the numbers in between. Adobe Stock 261656757
New Structures, Simulated and Studied with Bridges-2, Offer Novel Medical and Industrial Applications
Metal nanoparticles have been useful tools in applications as different as reducing pollution, treating disease, and creating flexible electronics. But scientists’ ability to predict the structure and activity of these tiny crystals has been limited. Using artificial intelligence (AI) and atomic simulations on PSC’s Bridges-2, a team from Pennsylvania State University has discovered over 20 nanoparticle structures not before predicted. These structures promise previously unknown chemical and physical properties that may be tuned to suit new applications.
WHY IT’S IMPORTANT
Metal nanoparticles are the most revolutionary tools that you may never have thought of. But the practical applications for these millionths-of-an-inch-sized metal dots are everywhere. As fuel additives, they can result in more efficient burning with less pollution. In medicine, they can kill bacteria without being toxic to us. They can also serve as microscopic monitors of health and disease. Added to asphalt, they can make roads more durable. Closely related metal nanowires can make touchscreens flexible, or help your clothes collect energy from your movements to recharge your smartphone.
Exactly what metal nanostructures can do depends on the shape of their outside surfaces. These surfaces depend a lot on the exact number of atoms in a given nanoparticle. Certain “magic” sizes lead to symmetrical shapes with different properties and uses. Even more interesting are the particles in between those sizes. These often amorphous — “shapeless” — structures offer the promise of new applications, but they’re way too complex for humans or computer simulations based on first chemical principles to predict.
“Metal nanoparticles have been around forever, as catalysts for chemical reactions. People have known for a long time that the activity or selectivity of a catalytic reaction is really sensitive to the surfaces that a particle would present. But what’s become new in more recent years is the ability to synthesize these particles with the best facets. What’s becoming more possible is the ability to characterize the surface structure … of very small particles.”
— Kristen Fichthorn, Penn State
That’s why Penn State’s Huaizhong Zhang, a graduate student working in the laboratory of Merrell Fenske, Professor of Chemical Engineering, and Professor of Physics Kristen Fichthorn, used PSC’s flagship Bridges-2 supercomputer to explore the structures of copper or silver nanoparticles with AI. The scientists got access to the system via the NSF ACCESS network of advanced computing centers, in which PSC is a leading member.
HOW PSC HELPED
When the team started their work, scientists had identified at least three categories of metal nanoparticles. In addition, a fourth had been predicted but not observed. The Penn State team expected that AI could detect more. But just having computer predictions wouldn’t be good enough. They wanted to understand why, to be able to dissect the AI’s decision making so that they comprehended what was going on — and why the predictions were valid — rather than just saying, “The computer said so.”
Another important question was whether a given particle would be an equilibrium structure or a kinetic structure. In the first, the particle’s atoms have settled into a stable state that will not change without external forces. In the second, the particle is merely at a waypoint in stability, from which it may, given time, settle further into a different state. For a particle that is small enough, it is possible that an equilibrium state won’t be reached, and the particle will forever change at some time scale.
The approach the Penn State scientists took involved first simulating the particles, and then, via a method called common neighbor analysis (CNA), creating signatures to classify the structures in a way that the AI could recognize and use to make predictions.
To achieve this, they needed serious computing power. The theorized particles contained 100 to 200 metal atoms, each of which needed to be simulated. Determining whether a particle had reached equilibrium would require 30 or more repeated simulations. And that was just the simulation part — the AI would then need to take those simulations and cross reference them with the known properties of the copper or silver atoms to explore millions upon millions of possibilities.
“[Bridges-2] is essential. We wouldn’t use Bridges if it wasn’t essential, because we have our own [computing] facilities at Penn State. Just to be able to pull these off, to be able to fit these force fields, takes Bridges’ extreme memory.”
— Kristen Fichthorn, Penn State
Bridges-2 was perfect for their mission. The system contains four extreme memory nodes, each of which contains 4 terabytes of RAM, together bringing 250 times as much memory as a high-end laptop to the job. It also offers 33 late-model, AI-friendly GPU nodes that would be needed to train the AI. Finally, Bridges-2 was designed from the ground up to trade large volumes of data between its nodes efficiently, so that computations like this that required different types of hardware for different steps could proceed quickly.
The AI’s predictions were eye opening. For both copper and silver, it predicted five major classes of nanoparticle. It further split each into subclasses, 14 for silver and 15 for copper. In each case, there were at least 10 types of nanoparticle that hadn’t been predicted by human analysis or simple simulations. Better yet, the CNA signatures of the new structures could be used to understand how they came about, lending confidence that the predictions were valid in a way that humans could understand. The team published their findings in the journal Nanoscale in August, 2024.
Next, the Penn State scientists are working on refining their predictions. Rather than just describe the shape of the particles, they want to use Bridges-2’s power to more accurately describe the interactions between individual atoms in the particle, by a process called machine learning force fields. A future paper will address this work.