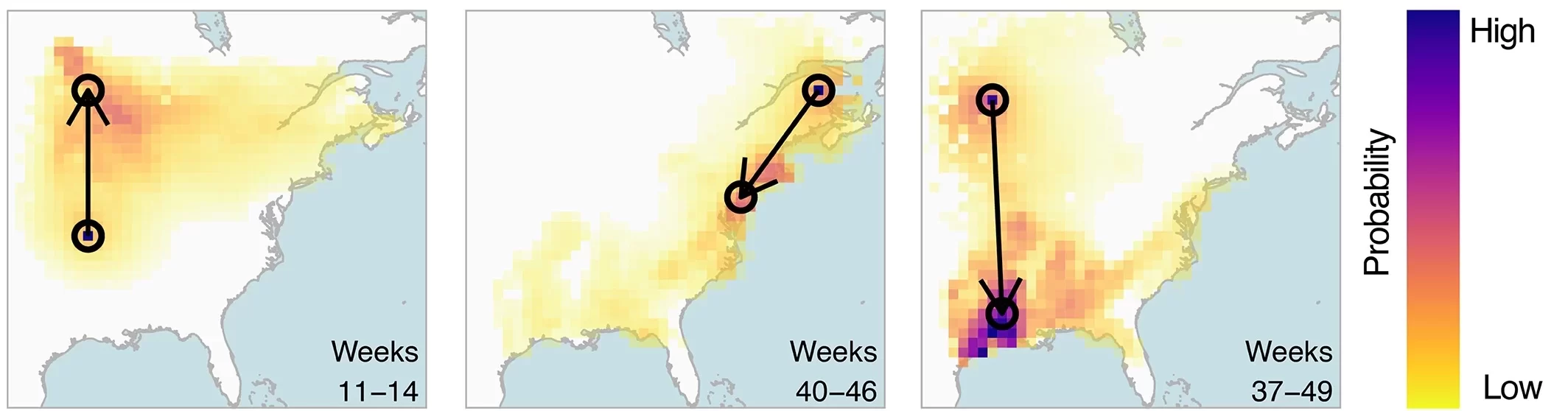
Predictions made by the BirdFlow AI on how the American woodcock migrates in North America between eBird “snapshots.” From Fuentes, M., Van Doren, B. M., Fink, D., & Sheldon, D. (2023). BirdFlow: Learning seasonal bird movements from eBird data. Methods in Ecology and Evolution, 14, 923– 938.
Bridges-2 Central in Removing Biases from Global eBird Database so AI Could Predict How Birds Travel
Migratory birds are critical for the health of human agriculture and the environment. But our knowledge of their movements is surprisingly little. Using “snapshots” based on Cornell University’s eBird database, scientists at the University of Massachusetts created BirdFlow, an artificial intelligence (AI) that accurately predicted migratory movements. Critical for the AI’s accuracy, the team first used PSC’s Bridges-2 system to remove observation biases from eBird and fill in gaps in the data.
WHY IT’S IMPORTANT
Migratory birds play an important role in our environment — and our dinner plates. By eating an astounding 400 to 500 million tons of insects each year, songbirds help protect the crops that humans and our domestic animals eat. By eating mice and rats, hawks protect our stored grain as well as reduce the number of disease-carrying ticks and rodent-borne pathogens. And that’s not even counting birds’ importance as indicators of environmental health.
It may surprise you, then, to hear that our information on bird migration is … hazy. That’s why scientists at Cornell University launched eBird, an online project to collect data on bird sightings by amateur enthusiasts worldwide. The project has given researchers a tidal wave of data on where certain birds can be found at what times of year. But the database only gives snapshots, not actual bird movements.
“The idea of bringing these sorts of methods to the problem of bird migration is something that Dan [Sheldon] has had on his mind for some time … The quality of the data and the access to computation had both improved pretty drastically since he first attempted the project many years ago.” — Miguel Fuentes, UMass
Miguel Fuentes, a graduate student in Daniel Sheldon’s group at the University of Massachusetts (UMass) Amherst teamed up with Benjamin Van Doren and Daniel Fink of the eBird team at the Cornell Lab of Ornithology to develop BirdFlow, an AI-based tool to fill in the gaps of eBird. They used PSC’s flagship, NSF-funded Bridges-2 supercomputer to handle the massive computation and data movement needed to prepare eBird data for the AI.
HOW PSC HELPED
As long ago as 2021, eBird had collected a billion bird observations, drawn from 77,466,000 checklists submitted by 684,300 volunteers in 202 countries. The data present a series of snapshots that, for all the world, look like stills from a video. But this view could be deceptive. Just because a type of bird is seen in one place on one date and a distant place a month later doesn’t mean that the species traveled between those two places — let alone that it’s the same bird.
Fuentes wanted to create an AI that could make informed guesses as to what happened in between the snapshots. But he’d need to validate its predictions somehow. Normally, a machine learning AI would use a fraction of a given data set to “train” itself. It would then make a series of trial-and-error guesses, correcting itself until its predictions were accurate. Then scientists would test its accuracy with the larger, whole dataset.
But the eBird data don’t directly record bird movements needed to evaluate the predictions. Instead, Fuentes would use the entire eBird data to train the AI — a new innovation. To test it, he’d use a much more definitive, if smaller, data set collected by another group of scientists: tracking data collected from individual birds’ migrations. By testing against real migration data from tagged birds, he could be sure that the AI’s predictions were valid.
The raw eBird data was not enough, though. Bird watchers aren’t evenly distributed around the world — in particular, they’re more likely to live in affluent countries. Also, the quality of the observations vary, based on the abilities of each individual volunteer. Before Fuentes could use the eBird data to train his AI, such biases would need to be corrected.
“Turning those very messy observations from lots and lots of participants into reliable information relies on a lot of computing.” — Daniel Fink, Cornell University
Fuentes could train and run his AI using the computing resources available to him at the UMass. But the Cornell scientists would need far more computing power for their data clean-up. For each of the 2,300 bird species they would analyze over the course of a year, they would need 2 to 8 gigabytes (GB) of computer memory and 3,000 to 4,000 CPU hours. In order to carry out the analysis in a reasonable amount of time, they’d need to run many species in parallel. Bridges-2’s “regular memory” nodes, offering 256 to 512 GB of memory apiece (enough to qualify as “large memory” on most supercomputers), gave them exactly this capability. By managing their use of memory carefully, they processed some 15,000 to 20,000 GB of data per month.
“We couldn’t do this without the scale of Bridges-2. Access to memory allocations is important too … [Also], the stability of Bridges-2 was better than any of the commercial options.” — Tom Auer, Cornell University
Early results are promising. BirdFlow on its own predicted migration patterns that matched the movements of the tagged birds well. Adding random movements, to simulate the uncertainty of individual birds’ courses, improved the match with the tracking data even more. The group reported an initial analysis of 11 species of North American birds in a paper in the journal Methods in Ecology and Evolution in January 2023.
In the cleaned-up eBird tool, and its application to BirdFlow, the scientists have created a group of resources that they would like to see other researchers use. You can see how eBird tracks observation snapshots here. The AI can suggest migration routes as well as their timing and connectivity in ways that biologists can then test in the field. The tool promises advances in many fields, including migration ecology, conservation, disease surveillance, aviation, and public outreach.