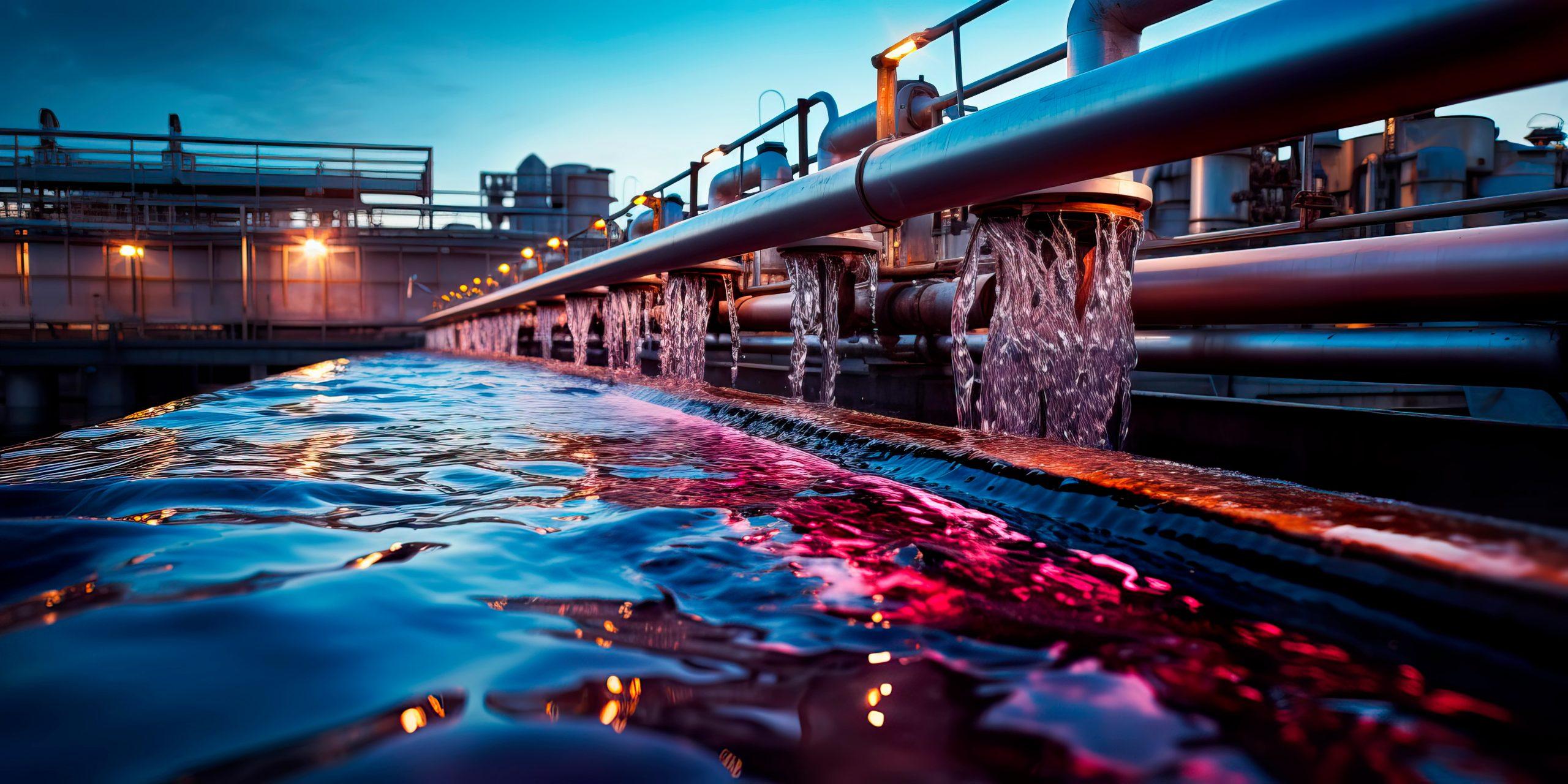
Adobe stock image: https://www.psc.edu/wp-content/uploads/2024/02/AdobeStock_646474337-scaled.jpeg
CMU Group Uses Bridges-2 to Create Virtual Plant to Learn from Veteran Engineers, and Next Train and Work with Rookies
The people who keep our drinking water safe are getting older. Soon they’ll retire. To avoid a crisis in the water-treatment industry, we need to train more experts. A team from Carnegie Mellon University’s Department of Civil and Environmental Engineering has used PSC’s Bridges-2 system to create a virtual water-treatment plant. The plant has enabled their artificial intelligence (AI) to learn from human expertise, by running veteran engineers through simulated breakdowns. They next hope to use this virtual-reality (VR) game to train new engineers. The end goal is an human-AI co-learning solution that both trains and works with water-treatment staff to leverage the best of machine and human intelligence.
WHY IT’S IMPORTANT
The U.S. is headed for a drinking-water crisis, and it has nothing to do with pollution, lead pipes, or population growth in the Southwest. Those things are all important, to be sure; but there’s another problem headed for us that isn’t getting the same number of headlines. The people who know how to keep our water safe are retiring. Water treatment plants across the country are now being run mostly by 60-somethings who won’t be working for much longer. Their expertise in the tricky business of keeping complex water purification systems up and running is about to timeout. The industry is also having trouble holding on to newcomers, who face a steep learning curve and can find good engineering jobs elsewhere.“The major problem is the difficulty to maintain drinking-water infrastructure with an aging workforce … What I observe is that here in the U.S., at least in Pennsylvania, we see in a lot of those water treatment plants that the average age is above 60.” — Pingbo Tang, CMU
HOW PSC HELPED
The problem was, to exaggerate a little, a “you can’t get there from here” situation. In order to work properly, a deep-learning AI needs to train itself on data where the “right answers” have been identified by human experts. But when you’re talking about a plant with many pumps and tanks with complex flows throughout, human experts work as much by feel and experience as by strict “when this happens, you do that” rules. Obviously, you can’t have the AI or a trainee experiment with a real plant the way they would on a simulator — but you can’t get to the simulator without learning how operators’ commands affect different problems in the system. The number of possible ways of getting things wrong can be infinite because of many combinations of malfunctions and human reactions that could influence each other.
The team started by simulating the plant’s physical parts. Bridges-2’s late-model GPUs, a technology originally designed to render high-quality images, were crucial for creating the plant, and its controls, inside the computer. Their next step was to create “problems” in the VR facility — and determine how to fix them. That’s where the human experts came in. By observing how the veteran engineers reacted to plant malfunctions that they’d faced in real life, the AI was able to learn how to spot where the issues were coming from and the sometimes not-obvious ways the old hands fixed it. Bridges-2’s GPUs, which are also particularly good at the pattern recognition necessary for machine learning, played a central role in that as well.
“… if we want to build the virtual reality environment, we need a lot of strong computing resources … We get a lot of human data, and if we want to train a large-language model, we [also] need strong computing resources … from PSC. [We need powerful] GPUs, for rendering the virtual reality environment, and also for training and testing the models.” — Pengkun Liu, CMU
The results of this initial work are promising. The computer’s classification recall for correctly identifying problems in the virtual plant was 0.712, with 1 being the perfect score. The human experts’ recall was 0.851. Better, combining the machine’s and peoples’ skills increased the recall to 0.885. The team presented their initial findings in a paper at the 2023 ASCE International Conference on Computing in Civil Engineering.
Now that they have a beta version of their VR water-treatment plant, they plan to put rookie engineers into it, having them play the simulated plant like a video game. By leveraging the experts’ input and what the AI learned from it, they can transition from the machine learning from humans to the machine teaching humans. In theory, the partnership could be a model for both training water-treatment engineers and running plants more effectively.
The work is, however, only a start. One major issue in the next step, training humans and getting the human and machine working together, is that different plants have different designs and complexity in real plant conditions that deviate from as-designed. AIs are great at what they’ve been trained to do, but they struggle to adapt as well as humans can. A larger plant, or one with a different design, may behave differently and require the AI to adapt in just such a way. The researchers are optimistic, though, that the good start they’ve made with Bridges-2 will help them get to that goal.