Neocortex
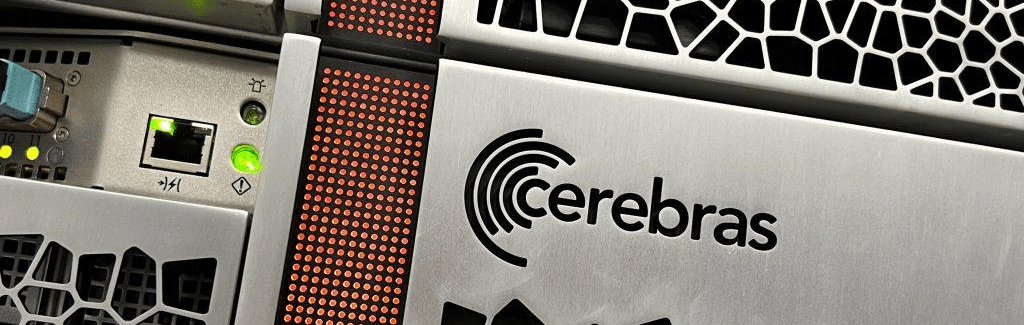
Cerebras CS-2, the world’s most powerful AI system, is the main compute element of Neocortex.
Unlocking Interactive AI Development for Rapidly Evolving Research
Neocortex is a highly innovative resource that targets the acceleration of AI-powered scientific discovery by greatly shortening the time required for deep learning training and by fostering greater integration of artificial deep learning with scientific workflows. The revolutionary hardware in Neocortex facilitates the development of more efficient algorithms for artificial intelligence and graph analytics.
With Neocortex, users can apply more accurate models and larger training data, scale model parallelism to unprecedented levels, and avoid the need for expensive and time-consuming hyperparameter optimization.
Is there a fee to use Neocortex?
There is no cost to use Neocortex for non-proprietary research. Users conducting proprietary research can find out more by requesting affiliation through the center’s Corporate Affiliates Program.
Is Neocortex a good fit for my research?
Four types of applications are supported on Neocortex. More detailed information on each type and what it involves can be found in the Neocortex documentation.
Choose any of the topics below to learn more about using Neocortex
Research projects
Research on Neocortex spans topics from NLP through physics, chemistry and biology. See some of the exciting projects using Neocortex to advance knowledge.
Getting an allocation
See how to apply, including eligibility requirements and the Neocortex Acceptable Use Policy.
Training
Training on the Neocortex system presents a system overview of this innovative platform for AI and ML research, and insights on how to best take advantage of its unique hardware, software, and capabilities.
User guide
Learn about the applications that are supported and how to conduct your research on Neocortex.
Questions? Contact us at neocortex@psc.edu.
System specifications
Neocortex features two Cerebras CS-2 systems and an HPE Superdome Flex HPC server robustly provisioned to drive the CS-2 systems simultaneously at maximum speed and support the complementary requirements of AI and HPDA workflows.
Neocortex is federated with PSC’s flagship computing system, Bridges-2, which provides users with:
- Access to the Bridges-2 filesystem for management of persistent data
- General purpose computing for complementary data wrangling and preprocessing
- High bandwidth connectivity to other ACCESS sites, campus, labs, and clouds
Cerebras CS-2
Each CS-2 features a Cerebras WSE-2 (Wafer Scale Engine 2), the largest chip ever built.
- AI processor:
-
Cerebras Wafer Scale Engine 2
- 850,000 Sparse Linear Algebra Compute (SLAC) Cores
- 2.6 trillion transistors
- 46,225 mm² 40 GB SRAM on-chip memory
- 20 PB/s aggregate memory bandwidth
- 220 Pb/s interconnect bandwidth
- System I/O:
- 1.2 Tb/s (12 × 100 GbE ports)
HPE Superdome Flex
- Processors
-
32 x Intel Xeon Platinum 8280L
- 28 cores, 56 threads each
- 2.70-4.0 GHz
- 38.5 MB cache
- Memory
- 24 TiB RAM, aggregate memory bandwidth of 4.5TB/s
- Local disk
-
32 x 6.4TB NVMe SSDs
- 204.6 TB aggregate
- 150 GB/s read bandwidth
- Network to CS systems
-
24 x 100 GbE interfaces
- 1.2 Tb/s (150 GB/s) to each Cerebras CS system
- 2.4 Tb/s aggregate
- Interconnect to Bridges-2
-
16 Mellanox HDR-100 InfiniBand adapters
- 1.6 Tb/s aggregate
- OS
- Red Hat Enterprise Linux
Acknowledgment in publications
Please use the following citation when acknowledging the use of computational time on Neocortex:
Buitrago P.A., Nystrom N.A. (2021) Neocortex and Bridges-2: A High Performance AI+HPC Ecosystem for Science, Discovery, and Societal Good. In: Nesmachnow S., Castro H., Tchernykh A. (eds) High Performance Computing. CARLA 2020. Communications in Computer and Information Science, vol 1327. Springer, Cham. https://doi.org/10.1007/978-3-030-68035-0_15
This material is based upon work supported by the National Science Foundation under Grant Number 2005597. Any opinions, findings, conclusions, or recommendations expressed in this material are those of the author(s) and do not necessarily reflect the views of the National Science Foundation.
Training
Neocortex Office Hours
Wednesdays, 2-3 EST. Learn more
Contact us
Email us at neocortex@psc.edu
Newsletter sign-up
Neocortex news & events
Fall Workshop: Accelerating Scientific Discovery with PSC’s Neocortex
This comprehensive 3-hour virtual workshop explained the Neocortex project, the technologies featured in the system, the type of applications supported (AI and non-AI), project highlights, demo sessions, and how to gain access.
ByteBoost Workshop: Experiential Learning on NSF Supported Computing Testbeds
Students from across the U.S. gathered at PSC in August to present their AI research projects as part of the 2024 ByteBoost Summer Workshop. These projects studied critical topics as diverse as engineering and discovering new drugs, understanding congressional policy outcomes, and safely controlling traffic for small, individually owned aircraft.
Neocortex Spring 2024 Workshop
Presented on March 29, 2024, by Mei-Yu Wang, PhD., Machine Learning Research Scientist, and Julian Uran, Machine Learning Research Engineer, of the Pittsburgh Supercomputing Center’s AI & Big Data Team.
This webinar was presented as a part of the ByteBoost program, and provides a system overview of Neocortex, an AI-specialized NSF-funded supercomputer deployed at PSC/CMU.
Neocortex Among Elite Artificial Intelligence Computers Selected for NAIRR Pilot Project
The Neocortex AI system is among six national AI supercomputers chosen to participate in the National Artificial Intelligence Research Resource pilot program to support novel and transformative AI research and education at a national scale. Neocortex was chosen for the NAIRR pilot project in part because of its innovative, AI-specialized design, meant to advance the state of AI beyond what was possible with the GPUs pioneered in the mid 2010s.
Neocortex Research Wins HPCWire Editor's Choice Award for Best HPC in Industry
Neocortex Overview for Campus Champions
This presentation gave an overview of Neocortex to the Campus Champions community.
NETL & PSC pioneer first CFD simulation on Cerebras Wafer-Scale Engine
Running on Cerebras CS-2 within PSC’s Neocortex, the National Energy Technology Laboratory simulated natural convection with multi-hundred million cell resolutions, pointing the way to more powerful, energy efficient and insightful scientific computing.
Webinar: Overview of Neocortex and upcoming CFP
This webinar presented an overview of Neocortex, in conjunction with the Spring 2023 Call for Proposals.
Webinar: Overview of Neocortex and upcoming CFP
This webinar presented an overview of Neocortex, in conjunction with the Summer 2022 Call for Proposals.
Neocortex upgraded to Cerebras CS-2 systems
The CS-2 systems are powered by the second-generation wafer-scale engine (WSE-2) processor. The WSE-2 doubles the system’s cores and on-chip memory and offers a new execution mode with even greater advantages for extreme-scale deep-learning tasks, enabling faster training, larger models and larger input data.
Webinar: Neocortex CS-2 overview
This webinar gave an overview of the recent Neocortex upgrade, now featuring two Cerebras CS-2 systems, in order to help researchers better understand the benefits of the new servers and changes to the system.
Webinar: Overview of Neocortex and upcoming CFP
This webinar presented an overview of Neocortex, in conjunction with the Fall 2021 Call for Proposals.
Neocortex Early User access begins
The Neocortex Early User Program provided a unique opportunity for the earliest possible access to the remarkable technology of the Cerebras CS-1 and HPE Superdome Flex integrated system. Participating research groups received one-on-one assistance from PSC and Cerebras experts to port, scale, and optimize their workflows.
Webinar: Getting Ready to Use the Neocortex System
In this hands-on virtual training, Neocortex Early User Program participants gained access to an environment where they could compile their code with Cerebras specific tools and produce metrics to inform the approach to optimally execute their code on the Cerebras CS-1 servers available in Neocortex.
Webinar: Technical Overview of the Cerebras CS-1
This webinar offered a technical deep dive into the Cerebras CS-1 system, the Wafer Scale Engine (WSE) chip, and the Cerebras Graph Compiler.
Webinar: Introduction to Neocortex
This webinar presented an overview of Neocortex, which captures groundbreaking new hardware technologies and is designed to accelerate AI research in pursuit of science, discovery, and societal good.
NSF Funds Neocortex, a groundbreaking AI supercomputer
A $5 million National Science Foundation award allows the PSC to deploy a unique high performance AI system. Neocortex introduces fundamentally new hardware to greatly speed AI research.